Understanding the Impact of Machine Learning and AI on Customer Service and Experience
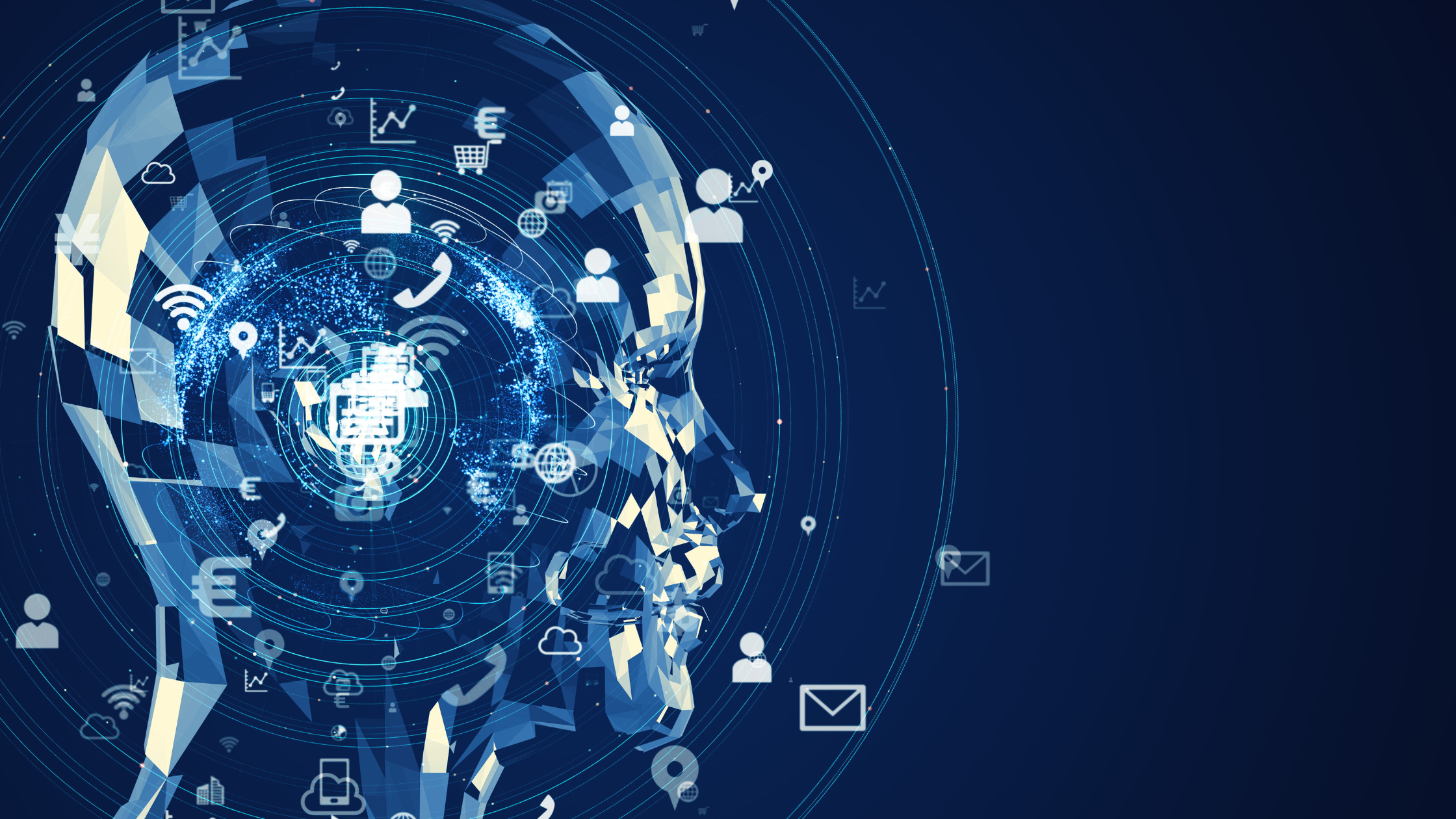
In today's fast-paced business environment, understanding customer behavior is crucial for delivering exceptional customer service. With the advent of machine learning (ML) and artificial intelligence (AI), businesses now have powerful tools to predict customer behavior, enhancing their ability to meet and exceed customer expectations. By providing actionable insights for individual customers, ML empowers businesses to tailor their services accordingly, revolutionizing the customer experience.
Generative AI: A New Era in Customer Service
Generative AI has significantly transformed customer service technologies, propelling us into a new era of automation. As outlined in our CEO Sally Hurley's Forbes article, our journey began with data analytics, where we gained invaluable insights into customer sentiment and preferences. The true revolution came with automation: generative chatbots, advanced voice, and video bots now engage in natural, meaningful conversations with customers. These technologies offer instant, personalized responses, enhancing customer satisfaction and efficiency. AI's potential in customer service extends beyond basic automation, allowing for improved policy revisions, training module creation, quality assurance, and internal tech support, thereby elevating the overall customer experience.
What is Machine Learning?
Machine learning is a subset of artificial intelligence that involves training algorithms to learn from and make predictions based on data. Unlike traditional programming, where specific rules are set for every task, machine learning algorithms develop practices by analyzing and learning from data. This process is akin to human learning, where continuous exposure to information leads to improved understanding and problem-solving capabilities.
There are various types of machine learning, including:
- Supervised Learning:
- Example: Email Classification System
- Description: A supervised learning model is trained on a labeled dataset of customer service emails. Each email is tagged with a label indicating its category, such as "Billing Inquiry," "Technical Support," "Product Feedback," etc.
- Application: When a new email arrives, the model can automatically classify it into the appropriate category, routing it to the right department or suggesting responses to customer service agents. This improves efficiency and ensures that emails are handled by the most suitable team.
- Unsupervised Learning:
- Example: Customer Segmentation
- Description: An unsupervised learning model is used to analyze a large set of unlabeled customer data, such as purchase history, browsing behavior, and demographic information.
- Application: The model identifies patterns and clusters customers into segments based on similarities in their behavior and characteristics. For instance, it might group customers into segments like "frequent buyers," "discount seekers," or "new customers."
- Benefit: This segmentation allows the company to tailor marketing strategies and customer service approaches to different groups, enhancing personalization and targeting.
- Reinforcement Learning:
- Example: Personalized Chatbot
- Description: A reinforcement learning model is deployed to manage interactions between customers and a chatbot. The chatbot is trained through trial and error, receiving feedback on whether its responses lead to successful resolutions or customer satisfaction.
- Application: The chatbot learns from each interaction, adjusting its strategies to improve over time. For example, it learns which questions to ask to diagnose a problem effectively or which tone and phrases result in better customer satisfaction.
- Benefit: This continuous learning process helps the chatbot become more effective and efficient, providing better customer service and improving the overall customer experience.
The Role of Machine Learning in Customer Service
Why is machine learning such a powerful tool for enhancing the customer experience? The answer lies in its ability to predict customer behaviors. Predictive capabilities are invaluable for anticipating customer needs and customizing products and services to meet those needs. For consumers, when machine learning is used responsibly, prediction helps mitigate the overwhelming amount of information we encounter daily. By leveraging machine learning to forecast which content is most relevant to each person, customers benefit from improved recommendations, reduced junk mail, minimal inbox spam, and superior search results, among other advantages.
Below HBR outlines how machine learning can be used to improve the customer experience.
Utilizing Data for Ongoing Enhancement
Continuous improvement is a hallmark of successful businesses, distinguishing them from their competitors by uncovering operational efficiencies, facilitating adaptation to changing market conditions, and fostering a culture that prioritizes innovation. AI can pinpoint common customer questions and issues, enabling businesses to devise solutions that proactively address customer needs before they arise. With the immense volume of customer data available today, AI becomes indispensable in efficiently extracting trends and identifying pain points. This allows businesses to focus on developing products, services, and communications that align with their customers' current and future needs.
Machine learning is embedded in many aspects of our daily lives, from recommendation systems on streaming platforms to predictive text on our smartphones. Here are a couple great examples of companies using machine learning to improve customer experiences.
Real-World Applications of Machine Learning
Machine learning is embedded in many aspects of our daily lives, from recommendation systems on streaming platforms to predictive text on our smartphones. Here are a couple of great examples of companies using machine learning to improve customer experiences:
- BMW: The more you drive an AI-enhanced BMW, the more it learns about you. The car uses machine learning to automatically adjust the systems and cabin experience for each driver.
- Disney: Guests at Disney parks use MagicBand wristbands as room keys, tickets, and payment. The wristband collects information on where the guests are in the park to recommend experiences and even route people around busy areas.
- American Express: AmEx detects fraud in real time by quickly analyzing millions of transactions to pinpoint which charges aren’t real. The quick service means customers can resolve the problem almost instantly.
- Burberry: Burberry’s luxury accessories are some of the most counterfeited in the world. The company uses image recognition to scan just a small section of a purse and determine its authenticity based on the pattern, texture, and weaving.
Challenges and Considerations
While machine learning offers significant advantages, there are challenges to consider:
- Data Privacy and Security: Ensuring customer data is protected is paramount.
- Accuracy and Bias: ML models must be carefully designed to avoid biases and ensure accurate predictions.
- System Integration: Integrating ML solutions with existing customer service systems can be complex and requires careful planning.
The future of machine learning in customer behavior prediction is promising. Advancements in algorithms will lead to even more accurate predictions. The use of AI and ML in predictive analytics will continue to grow, emphasizing the importance of ethical AI practices to ensure fair and unbiased outcomes.
By understanding and leveraging the power of machine learning and AI, businesses can significantly enhance their customer service and experience, ensuring they stay ahead in an ever-evolving market. For more insights, check out our CEO Sally Hurley's Forbes article.
More From VIPdesk